Synergies and Challenges: Unraveling the Intersection of Quantum Computing and Artificial Intelligence
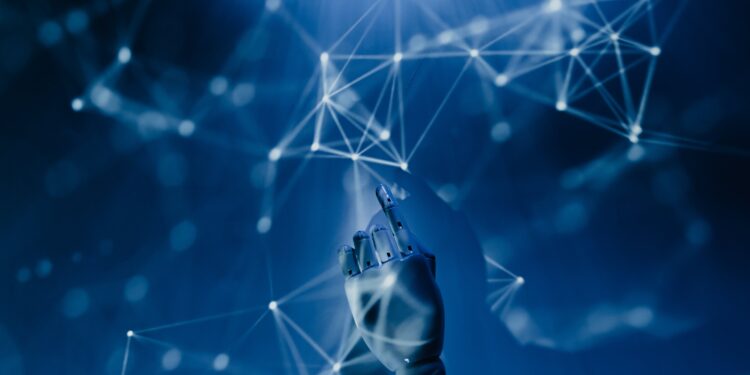
In recent years, the convergence of Quantum Computing (QC) and Artificial Intelligence (AI) has emerged as a cutting-edge domain with the potential to revolutionize computational capabilities. This topic explores the intricate relationship between quantum computing and AI, delving into the synergies, challenges, and transformative possibilities that arise when these two groundbreaking fields intersect.
Quantum computing, harnessing the principles of quantum mechanics, promises exponential speedup for specific computational tasks, surpassing the capabilities of classical computers. Simultaneously, artificial intelligence has witnessed remarkable strides, becoming an integral part of various industries. This intersection holds the promise of overcoming current limitations in AI computations, unlocking unprecedented efficiency and solving complex problems that were previously deemed intractable.
Synergies between Quantum Computing and AI:
- Optimized Machine Learning Algorithms: Quantum computing’s parallelism and superposition properties could be leveraged to optimize machine learning algorithms, leading to faster model training and more efficient optimization processes.
- Quantum Neural Networks: The development of quantum neural networks, which leverage quantum entanglement for enhanced information processing, could offer novel approaches to pattern recognition and decision-making in AI applications.
- Quantum-enhanced Data Processing: Quantum computers excel at processing vast datasets, potentially enabling more sophisticated analysis for AI applications that heavily depend on data, such as natural language processing and image recognition.
- Solving Optimization Problems: Quantum algorithms, like the Quantum Approximate Optimization Algorithm (QAOA), have the potential to address complex optimization problems, contributing to improved decision-making processes in AI systems.
Challenges and Considerations:
- Quantum Error Correction: Quantum computers are susceptible to errors due to environmental factors. Developing robust quantum error correction mechanisms is crucial to ensure the reliability of quantum computations in AI applications.
- Integration Challenges: Bridging the gap between quantum and classical computing poses integration challenges. Hybrid quantum-classical systems must be effectively designed to leverage the strengths of both paradigms.
- Access to Quantum Hardware: The current scarcity of stable and scalable quantum hardware poses a challenge to widespread adoption. Ensuring accessibility to quantum resources for AI researchers is crucial for advancing this interdisciplinary field.
- Algorithm Development: Tailoring algorithms to harness the unique features of quantum computing requires interdisciplinary collaboration between quantum physicists and AI experts. Developing quantum-enhanced algorithms that outperform classical counterparts is an ongoing research challenge.
The intersection of quantum computing and artificial intelligence opens up new frontiers, holding the potential to redefine the limits of computational power and problem-solving. While challenges remain, the synergies between quantum computing and AI present a fascinating avenue for exploration, paving the way for a future where quantum-enhanced AI systems drive innovation across various domains. Continued research, collaboration, and advancements in both fields are essential to unlock the full potential of this transformative convergence.